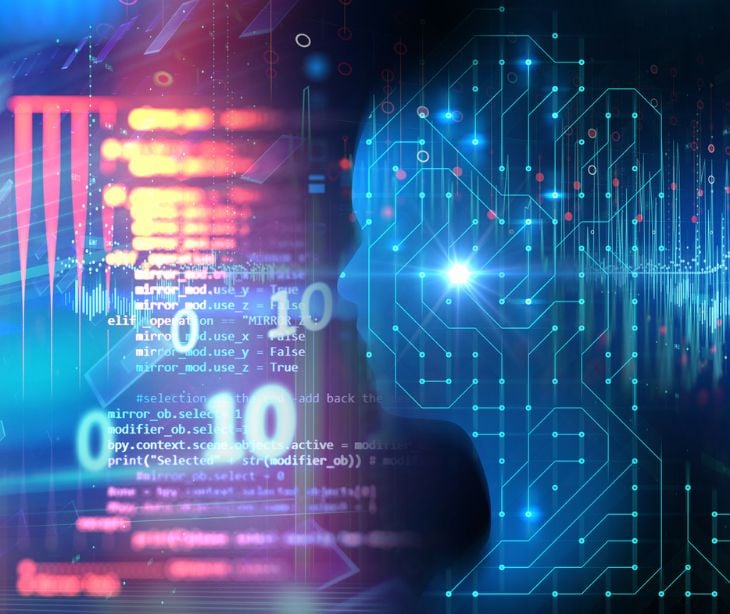
Under HIPAA, healthcare providers, health plans, and healthcare clearinghouses must adhere to strict standards when conducting data analysis involving patient information. Any research or analysis conducted on patient data must ensure that all personally identifiable information (PII) is de-identified and anonymized to prevent association with individual patients.
The goal is to strike a delicate balance between using patient data for research and analysis while respecting patient privacy rights and maintaining data security.
See also: Email AI: An evolving guide
Using AI in patient data analysis
By leveraging machine learning and deep learning algorithms, AI can identify patterns, trends, and correlations in patient data that may be challenging for human analysts to detect. This can lead to more precise and personalized diagnoses and treatment recommendations. AI-driven data analysis has the potential to improve patient outcomes, optimize treatment plans, and advance medical research. The methods of applying AI include
- Supervised learning: This method involves training AI models on labeled data where the desired output is known. For instance, AI can predict patient outcomes, diagnose diseases, or recommend personalized treatment plans based on historical patient data.
- Unsupervised learning: In this method, AI algorithms analyze unlabeled data to identify patterns, structures, and relationships without prior knowledge of the output. Unsupervised learning can cluster patients into different groups based on similarities.
- Deep learning: Deep learning is a subset of machine learning that utilizes artificial neural networks with multiple layers to process and analyze complex data. Deep learning models excel at image recognition tasks, enabling more accurate and efficient diagnoses in radiology and pathology.
- Natural language processing (NLP): NLP enables AI systems to understand and process human language in the form of clinical notes, medical literature, and patient interactions.
- Predictive analytics: AI-powered predictive models use patient data to forecast future health events, such as hospital readmissions, disease progression, or medication adherence. These models can help healthcare providers intervene proactively and optimize patient care.
- Drug discovery and genomics: AI is employed in drug discovery to analyze vast datasets and identify potential drug candidates. AI models can predict the effectiveness and safety of drugs based on genomic data and molecular interactions, accelerating the drug development process.
- Patient risk stratification: AI is used to assess patient risk by analyzing clinical, genetic, and lifestyle data. Risk stratification models help identify high-risk patients who may benefit from early interventions or targeted healthcare programs.
- Real-time decision support: AI can provide real-time decision support to healthcare professionals by analyzing patient data during medical consultations. This assists in making informed and evidence-based clinical decisions.
- Population health management: AI facilitates population health management by analyzing large datasets to identify health trends, disease outbreaks, and public health risks. It aids in designing targeted interventions and preventive measures to improve population health outcomes.
See also: HIPAA compliance and data analytics
Considerations to ensure that AI is used in a HIPAA compliant way
Before using patient data in AI applications, ensure that all personally identifiable information (PII) is removed or anonymized to prevent association with individual patients. This ensures that AI analysis is performed on de-identified data.
Furthermore, choose AI models that are explainable and transparent, especially in decision-making processes. Transparent AI algorithms help clinicians and healthcare professionals understand the reasoning behind AI-driven recommendations, building trust and acceptance.
Any AI model chosen should be assessed for any potential data-related biases, and IT staff should be in place to ensure that patient data is adequately assessed. If utilizing third-party vendors for AI solutions, ensure they are HIPAA compliant. Implement business associate agreements (BAAs) to hold vendors accountable for protecting patient data.
See also: HIPAA Compliant Email: The Definitive Guide
Subscribe to Paubox Weekly
Every Friday we'll bring you the most important news from Paubox. Our aim is to make you smarter, faster.