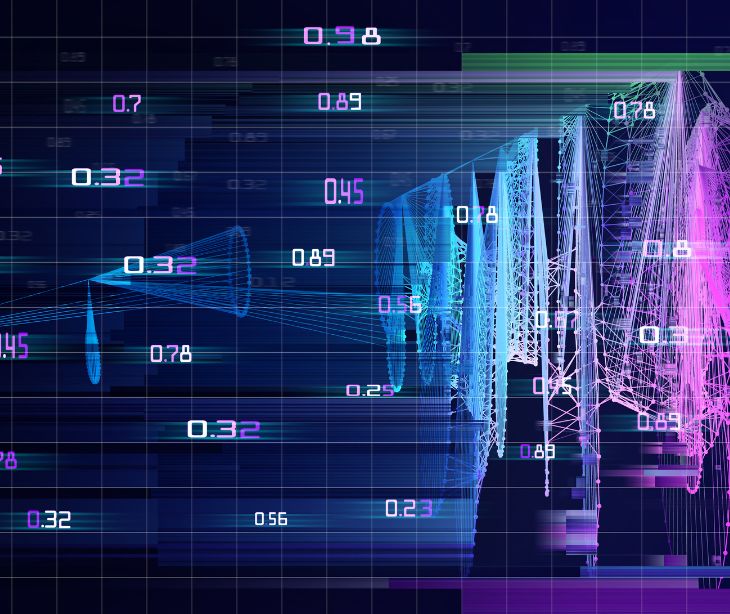
Healthcare algorithms are computational tools that analyze medical data to aid in clinical decision-making, diagnosis, and treatment planning. These algorithms, often powered by machine learning, process vast amounts of information from electronic health records (EHR) to identify patterns and make predictions about patient health outcomes. However, they are susceptible to various biases. One study titled, Dissecting racial bias in an algorithm used to manage the health of populations, revealed one of these biases, “The current use of algorithms that determine who receives access to high-risk health care management programs was found to routinely accept healthier whites into the programs ahead of less healthy blacks.” Biases like these can inadvertently reinforce existing healthcare disparities, particularly affecting minority and economically disadvantaged groups.
The potential biases in healthcare algorithms
Missing data: Inaccuracies arise when patient information is absent from healthcare records.
Sample size: Small or unrepresentative sample sizes can skew algorithm outcomes.
Misclassification: Incorrect categorization of patient data leads to faulty algorithmic conclusions.
Measurement error: Errors in data measurement and recording affect the reliability of algorithmic predictions.
Socioeconomic: Disparities in healthcare access and quality among different socioeconomic groups lead to biased data.
Implicit bias of healthcare providers: Prejudices and assumptions held by healthcare professionals can influence data input and interpretation.
Data representation: Algorithms might be biased if the data doesn't adequately represent diverse patient populations.
Algorithmic: The inherent design and function of the algorithm itself might be biased.
Overfitting to majority populations: Algorithms overly tailored to majority groups can fail to predict outcomes for minority groups accurately.
Underrepresentation of minority groups: Insufficient representation of minority groups in data sets leads to less accurate or relevant predictions for these groups.
See also: HIPAA Compliant Email: The Definitive Guide
What contributes to the existence of potential biases
Healthcare providers and algorithm designers help in creating biases in healthcare algorithms. Through their documentation practices, providers contribute to biases when they inadvertently omit patient information or when their implicit biases influence how they record data. These biases are often a result of personal experiences, training backgrounds, and subjective judgments. For example, socioeconomic or racial prejudices can lead to differential treatment and documentation for various patient groups, resulting in misclassification or measurement error biases.
On the other hand, the designers of these algorithms contribute to biases primarily during the development phase. Their choices in selecting, processing, and interpreting data can introduce algorithmic biases. For instance, if the training data predominantly features one demographic group, the algorithm may become overfitted to that group, neglecting the needs and characteristics of minority populations.
See also: Artificial Intelligence in healthcare
The impact of potential biases in healthcare algorithms
When algorithms used in healthcare fail to consider the diversity of patient populations, it becomes difficult for healthcare organizations to provide effective treatment for a wide range of people. This can lead to differences in health outcomes, impacting patient satisfaction and trust. It can also result in legal and ethical implications for healthcare organizations. Additionally, if healthcare organizations rely on biased algorithms, then their reputation may be at risk, as they could be perceived as unfair or discriminatory in their service delivery.
How guiding principles address potential biases
In response to the growing concern over potential biases in healthcare algorithms, especially regarding their impact on racial and ethnic disparities, a comprehensive effort is underway to address these issues. A recent paper in JAMA Network Open discusses steps taken by a panel of researchers from the Agency for Healthcare Research and Quality (AHRQ) and the National Institute for Minority Health and Health Disparities at the National Institutes of Health (NIH). They convened to establish guiding principles aimed at mitigating and preventing these biases. These principles emphasize fostering equity throughout the healthcare algorithm's life cycle, ensuring clarity and understandability of algorithms, involving patients and communities in all phases, addressing fairness issues explicitly, and implementing a system of accountability for equitable results.
See also: Guiding principles address biases resulting from algorithms
FAQs
What is the job of the NIH?
The National Institutes of Health (NIH) is responsible for conducting medical research and providing funding for research in various health-related fields to improve public health.
When do AI systems have to be HIPAA compliant?
AI systems must be HIPAA compliant when they handle, process, or store protected health information (PHI) for entities covered by HIPAA, such as healthcare providers or insurance companies.
Is separate consent necessary for patient data to be filtered through experimental AI systems?
Yes, separate consent is usually necessary for patient data to be used in experimental AI systems, especially if the data will be used in ways not covered by the initial consent for treatment or care.
Subscribe to Paubox Weekly
Every Friday we'll bring you the most important news from Paubox. Our aim is to make you smarter, faster.