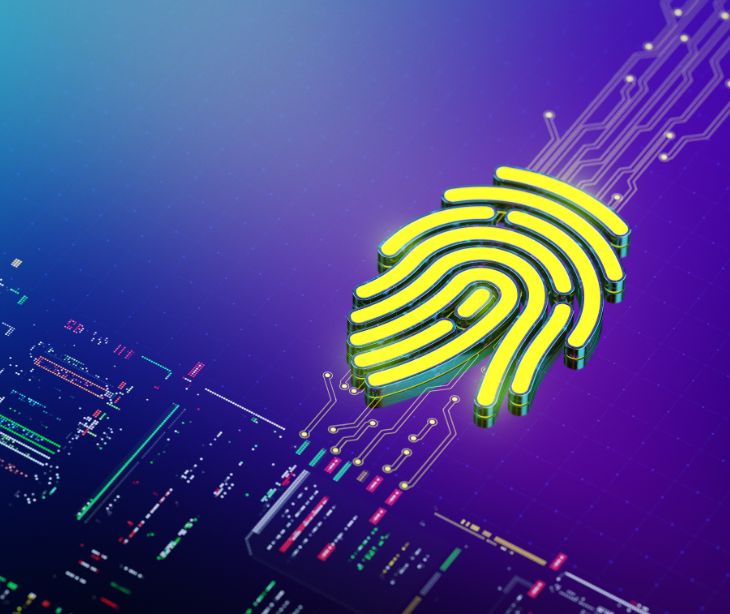
In biometric authentication, liveness detection is a technique used to distinguish between live human beings and fake representations. By analyzing data collected from biometric sensors, algorithms determine whether the source of a biometric sample is genuine or a spoof.
Understanding liveness detection
Liveness detection enhances the security of biometric systems. Differentiating between live human beings and fake representations prevents fraud, improves security, and protects user privacy.
With techniques such as motion analysis, 3D depth sensing, texture analysis, challenge-response tests, and machine learning AI, liveness detection algorithms are becoming increasingly sophisticated.
Related: What is advanced threat detection?
Types of liveness detection
Liveness detection can be categorized into two main types:
Active detection
Active liveness solutions primarily focus on detecting signs of life. These solutions prompt users to perform actions that are difficult to replicate with a spoof.
For example, facial recognition systems may ask users to blink, smile, or nod their heads. By analyzing the natural, involuntary movements of genuine users, active liveness algorithms can differentiate between live individuals and static images or videos. However, these methods often suffer from usability issues, lower accuracy rates, and require user interaction.
Passive detection
In contrast, passive liveness algorithms primarily aim to detect signs of a spoof but can also passively analyze signs of life. These algorithms do not require active participation from users, making them more user-friendly and highly usable. They can unobtrusively employ multiple algorithms, adapting to different client environments over time.
Benefits of liveness detection
Liveness detection is significant for several reasons:
Fraud prevention
Without liveness detection, biometric systems are vulnerable to attacks using high-quality photographs or videos. Liveness detection helps prevent such fraudulent attempts by verifying the authenticity of biometric data.
Enhanced security
Liveness detection strengthens the overall security posture of biometric systems by adding an extra layer of security. This makes them more reliable for applications such as mobile banking, access control, and secure authentication.
Privacy protection
Liveness detection ensures that only genuine biometric data necessary for identification is captured. This reduces the risk of privacy violations by using unauthorized materials or images.
Go deeper:
Techniques used in liveness detection
Liveness detection employs various techniques and technologies to differentiate between live human beings and spoofs. Here are some common methods:
Motion analysis
Motion analysis relies on tracking and analyzing the movement of the subject. For instance, facial recognition systems may instruct users to blink, smile, or nod their heads. Genuine users naturally respond with involuntary movements that can be detected, while static images or videos cannot replicate these movements.
Texture analysis
Texture analysis involves examining the fine details and textures of the subject's skin or fingerprint. Genuine skin exhibits unique features and perspiration patterns that are challenging to replicate with a photo or synthetic material. By analyzing these textures, liveness detection algorithms can distinguish between real and fake samples.
Challenge-response tests
Liveness detection may also include challenge-response tests, where the system asks the user to perform specific actions such as turning their head or speaking a random phrase. The system then analyzes the responses to determine liveness.
Machine learning AI
Powerful machine learning approaches utilizing advanced AI techniques have revolutionized liveness detection. These sophisticated algorithms can detect subtle image transformations that result from presentation attacks. They can infer 3D structures and identify subtle textural differences in spoofs that are invisible to the human eye.
Subscribe to Paubox Weekly
Every Friday we'll bring you the most important news from Paubox. Our aim is to make you smarter, faster.